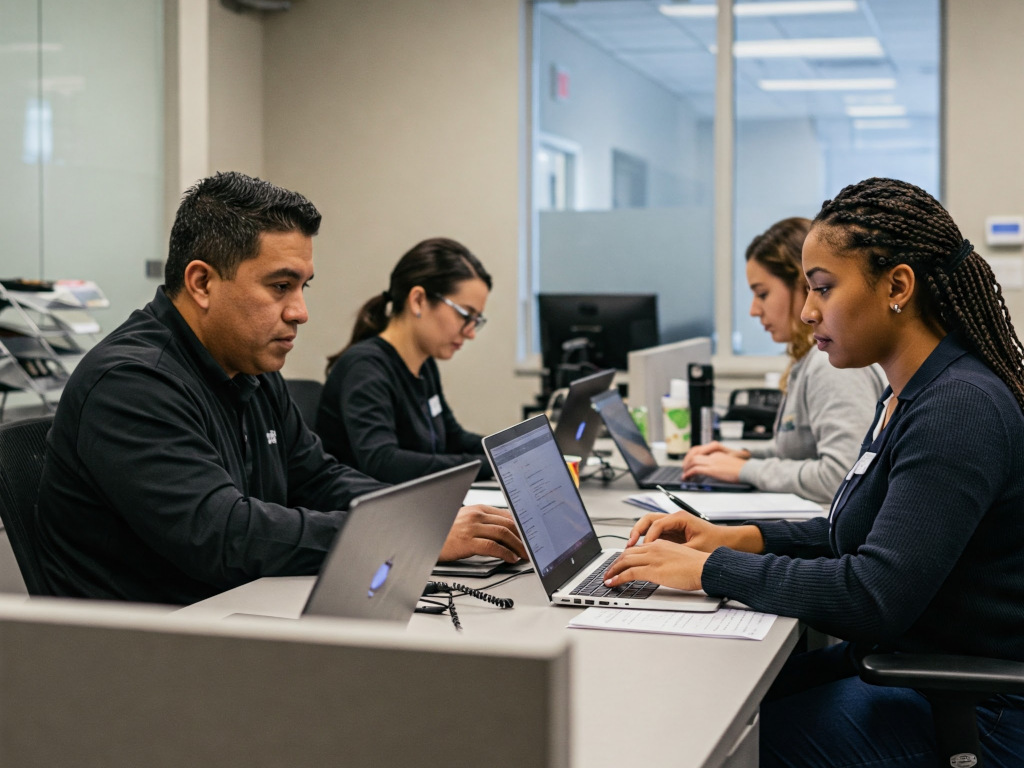

We help your business stay ahead of evolving fraud patterns, combining cutting-edge technology with industry knowledge to protect your organization from financial and reputational harm.
Our founder, David Lucker, spent several years combating fraud at scale in the high-risk ride-hailing and food-delivery industries. We leverage this experience to deliver best-in-class fraud detection and prevention solutions—tailored to your unique risks and built to protect you from both known and emerging threats.
Some examples in action:
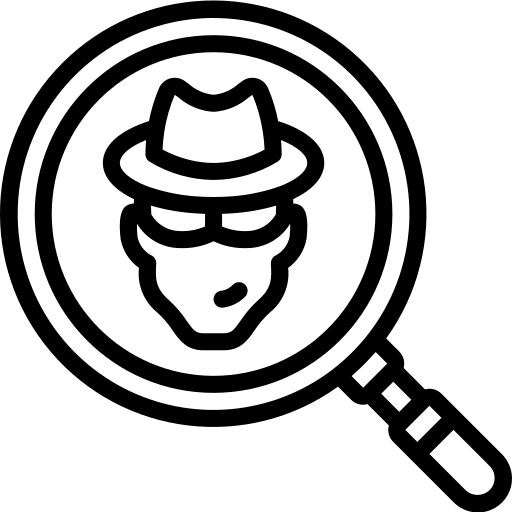
Implementation of essential fraud detection rules - We set up, maintain, and monitor fundamental fraud detection rules based on defined conditions, such as transaction frequency, repetitive patterns, or transaction timing. Before deployment, we run rules in shadow mode to validate their effectiveness and monitor key metrics like the false-positive rate.
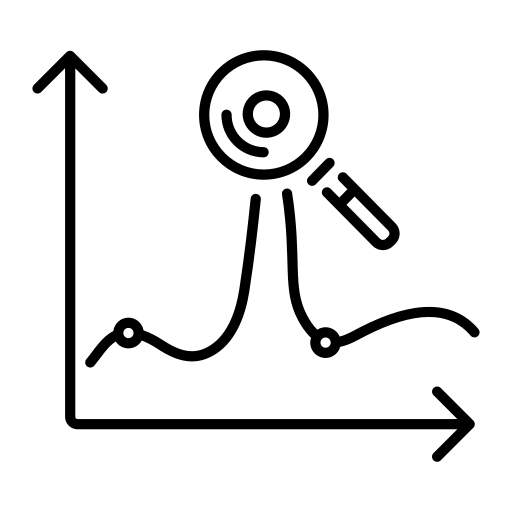
Anomaly Detection System – We implement a sophisticated anomaly detection system designed to uncover unusual patterns in your transaction data. The system first uses outlier detection to flag individual data points that deviate significantly from expected behavior. It then applies multidimensional clustering to group similar outliers together, making it easier to prioritize investigations and take targeted action. This layered approach enables the detection of complex fraud scenarios—including previously unseen or evolving fraud patterns—across a variety of use cases, such as financial fraud or incentive abuse in e-commerce environments.
Here is the full list of our services:
Rules-Based Detection
- Rule Development – Develop and deploy robust, rules-based logic tailored specifically to your business requirements.
- Monitoring & Optimization – Continuously monitor and optimize rules, ensuring minimal false positives and disruption.
- Ongoing Maintenance – Maintain and update detection rules regularly to address evolving fraud threats.
Unsupervised Machine Learning (ML)
- Emerging Fraud Patterns – Apply clustering, anomaly detection, and outlier analysis to identify previously unseen or emerging fraud patterns.
- Behavioral Anomalies – Detect subtle and unusual behaviors early, reducing exposure to new types of fraud.
Supervised Machine Learning (ML)
- Model Development – Utilize neural networks and graph-based methods trained on labeled datasets to precisely detect known fraud patterns.
- Feature Engineering – Conduct thorough feature engineering to enhance detection accuracy, precision, and recall.
- Large-scale Deployment – Deploy models at scale, suited for large operations with significant data volume.
Real-time and Batch Detection
- Flexible Systems – Provide flexible detection systems, configurable for both real-time responses and batch processing, depending on operational needs.
- Shadow Mode Testing – Run detection systems in shadow mode initially to safely evaluate effectiveness without impacting live operations.
Human Validation Integration
- Team Setup – Set up and train human validation teams to efficiently review flagged cases and manage fraud appeals.
- Workflow Design – Design optimized workflows that integrate human judgment seamlessly with automated systems.
- Feedback Loops – Establish feedback loops to improve automated detection based on human review outcomes.
Appeals Team Integration
- Appeals Learning – Connect fraud detection systems directly to appeal team outcomes, enabling models to continuously learn from false positives.
- Enhanced Accuracy – Enhance detection accuracy by systematically incorporating appeal insights into detection logic.
Strike and Sanction Systems
- Escalating Sanctions – Implement strike-based approaches where repeat offenses lead to escalating sanctions (temporary suspensions to permanent bans).
- Automated Communications – Automate communication to users about strikes, sanctions, and appeals processes, improving transparency and compliance.